The Evolution of Prompt Engineering: Time to forget what you have learnt?
Created on 13 September, 2024 • AI News & Insights • 207 views • 4 minutes read
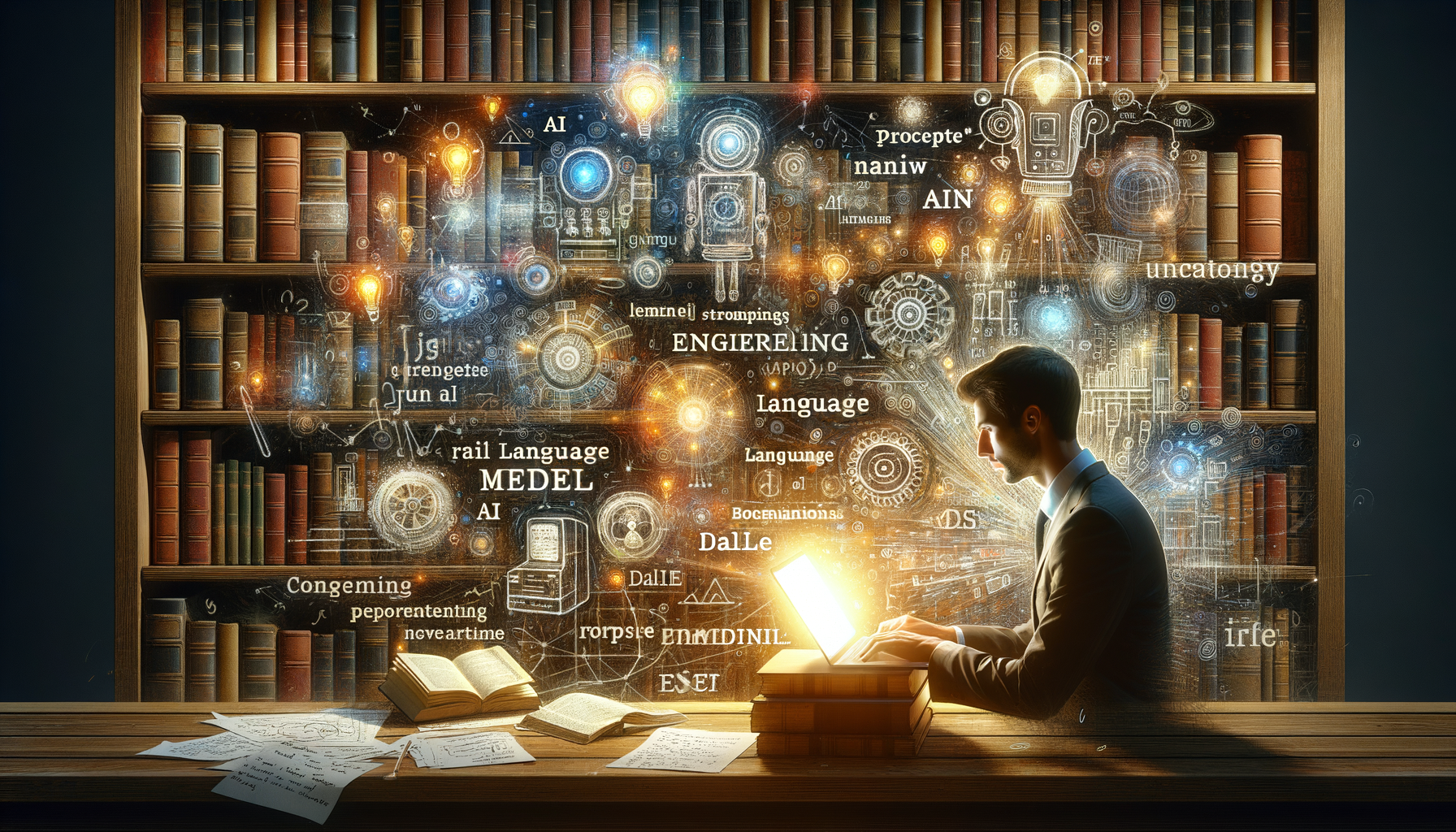
In this insightful blog post, we delve into the fascinating field of prompt engineering and how it has evolved over time. We'll examine the emergence and significance of advanced models like O1-Preview, and how they are revolutionizing everything.
Introduction
Prompt engineering has played a pivotal role in the utilization of language models, especially in bridging the gap between user intent and machine understanding. Traditional techniques have emphasized the importance of crafting detailed prompts, providing ample context, and employing methods like few-shot prompting to guide models toward desired outputs. However, with the advent of more advanced models like O1-Preview, these established practices are undergoing significant transformations. This article explores how models like O1-Preview are impacting prompt engineering and what changes end-users should expect in future interactions.
The Foundation of Prompt Engineering:
Before delving into the impact of advanced models, it's essential to understand why prompt engineering became crucial:
1. Detailed Prompts and Context: Early language models required explicit instructions to perform tasks effectively. Users had to meticulously craft prompts, ensuring that the model had all the necessary information to generate accurate responses.
2. Few-Shot Prompting: By providing examples within the prompt (few-shot learning), users could demonstrate the desired output format or style, helping the model to produce better-aligned responses.
3. Control over Output: Detailed prompting gave users a degree of control over the model's output, reducing instances of irrelevant or incorrect information.
These techniques were instrumental in harnessing the capabilities of earlier models, compensating for their limitations in understanding context and producing coherent, task-specific outputs.
Introducing Advanced Models Like O1-Preview
O1-Preview represents a new generation of language models that exhibit significant advancements in understanding, context retention, and response generation. Key features of these models include:
1. Enhanced Contextual Understanding: Advanced models can comprehend and retain context over longer interactions, reducing the need for repetitive or overly detailed prompts.
2. Improved Natural Language Processing: They better understand nuances, idioms, and indirect instructions, allowing for more natural and conversational interactions.
3. Reduced Dependency on Few-Shot Examples: With extensive pre-training, these models often require fewer examples to perform specific tasks effectively.
4. Adaptive Response Generation: They can adjust their responses based on subtle cues in the prompt, providing more relevant and accurate information.
Impact on Established Prompt Engineering Practices
The advancements in models like O1-Preview are reshaping how users approach prompt engineering:
1. Simplification of Prompts:
- Less is More: Users no longer need to craft excessively detailed prompts. A concise, clear instruction can yield high-quality responses.
- Natural Language Instructions: Prompts can be more conversational, reducing the need for rigid, formal structures.
2. Reduced Need for Few-Shot Prompting:
- Intrinsic Understanding: The models' enhanced capabilities diminish the necessity for providing examples within the prompt.
- Focus on Task Description: Users can focus on describing the task rather than demonstrating it through examples.
3. Enhanced Flexibility:
- Adaptability: Models can handle a wider range of tasks without explicit instructions, adapting to the user's intent more effectively.
- Contextual Continuity: They maintain context over longer dialogues, enabling more dynamic and interactive sessions.
4. Improved Response Accuracy:
- Error Reduction: Advanced comprehension leads to fewer misunderstandings and more accurate responses.
- Relevant Output: Models are better at filtering out irrelevant information, providing outputs closely aligned with user intent.
Changes End-Users Should Expect in Future Prompting
As models continue to evolve, users can anticipate several changes in their interaction with these systems:
1. Streamlined Communication:
- Ease of Use: Interactions will become more intuitive, requiring less effort in crafting prompts.
- Conversational Interfaces: Users can engage in more natural dialogues, similar to speaking with a human assistant.
2. Emphasis on Intent Over Instruction:
- Implicit Understanding: Users can rely on the model to grasp their intent without needing exhaustive explanations.
- Focus on Objectives: The primary concern will shift to what the user wants to achieve rather than how to instruct the model to do it.
3. Personalization and Context Awareness:
- Tailored Responses: Models may use prior interactions to personalize responses.
- Context Retention: The ability to remember and reference earlier parts of the conversation enhances continuity.
4. Reduced Learning Curve:
- Accessibility: New users will find it easier to get meaningful outputs without deep knowledge of prompt engineering techniques.
- Democratization of AI Interaction: Broader user base can effectively use AI tools without specialized skills.
Practical Examples
*Example 1: Task Completion Without Detailed Prompting*
Traditional Prompt:
```
Translate the following English sentence to French: "The quick brown fox jumps over the lazy dog."
```
With Advanced Model:
```
Translate to French: "The quick brown fox jumps over the lazy dog."
```
*The advanced model accurately performs the translation without needing the detailed instruction.*
*Example 2: Summarization with Minimal Prompting*
Traditional Prompt:
```
Summarize the following article in 2-3 sentences, focusing on the main points and omitting minor details.
[Article text]
```
With Advanced Model:
```
Please summarize this:
[Article text]
```
*The advanced model provides a coherent summary, understanding the request even with a brief prompt.*
Conclusion
The emergence of advanced language models like O1-Preview is set to revolutionize the field of prompt engineering. As these models become more proficient at understanding user intent and context, the need for elaborate prompting techniques diminishes. End-users can look forward to more accessible, efficient, and natural interactions with AI systems, focusing on their objectives rather than the intricacies of prompt construction.
The future of AI interaction lies in seamless communication, where the technology adapts to the user rather than the other way around. By embracing these changes, users can leverage the full potential of advanced models, unlocking new possibilities in productivity, creativity, and innovation.
Recommendations for Users
1. Experiment with Simpler Prompts: Try using straightforward language to communicate tasks and observe how the model responds.
2. Engage in Dialogues: Treat interactions as conversations, providing feedback and additional information as needed.
3. Focus on Clarity: While brevity is beneficial, ensure that your requests are clear to avoid ambiguity.
4. Stay Informed: Keep up with developments in AI models to understand new capabilities and adjust your approach accordingly.
By adapting to these evolving technologies, users can enhance their interactions with AI models, leading to more effective and satisfying outcomes.
Popular posts
-
About TeachBuddyTutorials • 437 views
-
-
-
-